Improve Real-Time Decision Making with Real-Time Supply Chain Data
Last Updated May 29, 2019
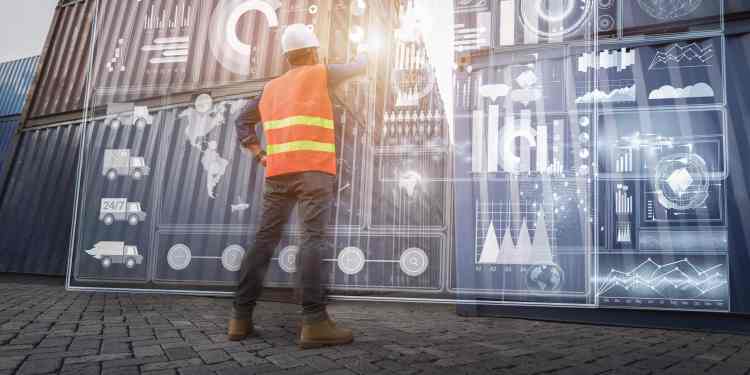
Today’s supply chains span continents and oceans, making end-to-end visibility both a challenge and a necessity. As the supply chain has gone global, technological innovations have essentially made the supply chain’s world smaller.
From cloud-based management systems to smart sensors, the real-time supply chain provides a constant stream of real-time data. This has enabled greater visibility, agility and in-the-moment decision-making via artificial intelligence (AI) and machine learning applications and data analysis and predictive analytics tools.
Secure greater visibility within your supply chain when you tap into and apply the real-time data of these digital systems, processes and applications:
Cloud-Based Solutions
Previously, organizations had to employ system administrators at the local network level to handle data storage networks. By adopting a cloud-based supply solution, storage is dispersed across all users, spreading the costs across locations and creating redundancies (a more effective “failsafe”) that can prevent costly downtime or data loss.
This full connectivity also enables better upfront planning. As organizations have more immediate access to data to power their decisions and scale more easily, new locations simply need to be incorporated instead of established from scratch.
One specific application is a transportation management system (TMS), previously only available as on-premise software. TMS has moved into the cloud, unlocking real time communication from shippers to carriers to partners and customers. TMS manages route planning, audits freights and payments, ensures order visibility, and manages carriers in a single platform. With more robust data, organizations can better consolidate shipping loads, select the lowest cost shipping methods, and improve routes with multiple stops, all steps that can directly cut costs.
Smart Sensors
The cloud isn’t just connecting links in the supply chain; smart sensors placed to specific machines at critical points along the supply chain can enable real-time data-gathering on site and at an individual item level – as opposed to previous methods that required decision makers to siphon through monthly or even quarterly reports. These sensors can then feed information to the cloud, permitting immediate monitoring and operations coordinating, insight into service logistics, and maintenance.
In the retail sector, this item-level data offers unprecedented insight into and control over inventory, both to better understand and manage backstock to avoid costly overages and to verify returned and received items are efficiently and accurately loaded. This can also help improve customer satisfaction, and even a shipping delay can be reported early to customers to keep them informed.
Across industries, when business decision makers are armed with real time reports, they can anticipate bottlenecks or breaks in the chain before they happen, instead of reacting to reported delays after they have already occurred.
Autonomous Warehouses
Thanks to Internet of Things (IoT)-equipped machinery and AI-powered robotics in industrial processing, big data is prolific. In many cases, the new challenge lies in harnessing the constant flow of real-time data to guide warehouse functions and in-the-moment decisions. Thanks to predicative analytics, this doesn’t have to stop with a sea of red flags for human management to troubleshoot.
Instead of leaning on supply chain managers to scramble to respond to the alerts raised by the system when something along the supply chain goes out of programmed bounds (for example “The delivery is late!”), AI-equipped machine learning can apply predictive analytics to estimate what will happen in the future. This moves supply chain management from reacting to data – even if it is real time – to responding to and planning for a set of future outcomes. As Forbes explains, this sea of data can continue to swell as all participants in the supply chain share their data and bolster the machine learning, making this smarter supply chain management beneficial to everyone in the system.
AI-Powered Demand Forecasting
While estimating demand has always been integral to supply chain management, businesses have historically solved for demand by overstocking items, an approach that tacks on costs in operations and storage. More precise demand forecasting can cut costs. Machine learning enables real-time analytics, adapting regularly to new information and allowing business to run leaner, without missing out on demand.
Organizations are turning to machine learning to help adapt and update forecasts in real time, with the hope that this agile, data-driven approach will reveal new ways to cut costs in operations and boost revenue. For example, computer manufacturing giant Lenovo partnered with IBM to employ AI to mitigate supply chain disruption, a key challenge for a global computer manufacturer in a highly competitive market. Lenovo applies AI to its risk management, which has drastically reduced the organization’s response to supply chain disruption – from days to minutes according to company estimates.
Not only does prolific access, improved flow, and increased visibility from real-time supply chain data better equip organizations to stay ahead of issues, it also enables businesses to plan for challenges or disruptions before they can even occur. For supply chain professionals to leverage this data technology to their competitive advantage, a clear understanding of the transformed landscape of supply chain management and an appreciation for adaptability is critical.